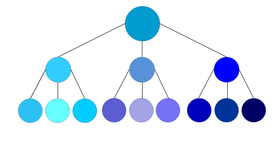
Structure Learning and Inference
Although there has been a wealth of research on causal inference, much of it has focused on only one particular form of causality, for instance, stimulus A leads to outcome B but not outcome C. Real-world learning and inference are much more complex. Many natural systems come in richer, structured forms, such as hierarchies (e.g. social groups), clustered environments (e.g. foraging patches), tree structures (e.g. sequential events), and cyclic events (e.g. seasons), and making inferences in these systems can leverage rich task representations that capture structural knowledge.
Our lab uses combinations of behavioral, computational, neuroimaging, and intracranial recording techniques to investigate how the brain forms, updates, and leverages such representations of the structure of a task or the environment to generalize and perform novel inferences. One current focus in the lab is how people abstract cognitive maps of relationships between entities or states in tasks (e.g. social networks, hierarchically related associations, hierarchical plans, etc.) to make efficient inferences, generalize to novel situations, and make flexible decisions.
Despite recent progress, there is still limited understanding of the neuronal mechanisms that underlie updating, inference, and generalization in structured environments. Such an understanding is central to any mechanistic description of human cognition and behavior in the real world. The importance of this is underlined by the fact that abnormal learning and generalization are likely to be core impairments in certain expressions of anxiety disorders and psychosis. Shedding light on the associated neural mechanisms thus holds promise for generating novel insights into the neural basis of these devastating disorders.