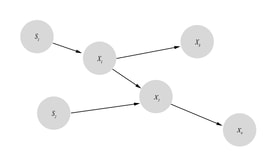
Causal Learning
What caused an illness, was it a specific loaf of rye bread or all gluten products? Why did it rain today and is this best explained by the cloud formation, time of year, or both? Such inference problems pervade our daily lives, and solving them adaptively has been critical for survival in our evolutionary past. All humans–from children to scientists–have an exceptional ability to detect and leverage structure present in the world. This capacity dramatically reduces the computational demand imposed by the stream of sensory information we receive daily by enabling us to abstract meaningful information and facilitating generalizations to entirely new situations that share similar structural properties.
Our labs use a combination of behavioral, computational, functional brain imaging, and intracranial recording techniques to investigate the neural representations and systems critical for causal learning, as well as the computations that underlie updating beliefs about causal relationships. This research topic spans forming and updating predictions about reinforcement (primary and secondary rewards), social outcomes (others' beliefs or rewards), and task states (stimuli or hidden contexts). A current focus in the lab is how the brain assigns credit - or attributes causal responsibility - to specific likely causal choices or events, exploring both 'model-free' and 'model-based' forms of credit assignment.